Closing the Gap: Challenges and Solutions for AI in the Real World
- Sunil Verma
- Apr 4, 2023
- 3 min read
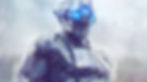
Artificial intelligence (AI) is no longer just a buzzword in the technology industry. In recent years, AI has made significant strides in transforming various sectors of the economy. AI is not only changing the way we work and live, but it is also creating new opportunities for businesses to improve efficiency, reduce costs, and increase profitability. However, there is a considerable gap between the AI capabilities that are being developed in academic and research settings and the AI applications that are being used in the real world. To bridge this gap, there is a need for AI that can operate in real-world environments, with real-world data, and real-world constraints. In this article, we will explore the challenges of applying AI in the real world and the potential solutions to address these challenges.
One of the main challenges of applying AI in the real world is the lack of high-quality data. Most AI algorithms require vast amounts of data to train and learn from, and the quality of the data is crucial in determining the accuracy and reliability of the AI model. However, real-world data is often incomplete, noisy, and unstructured, making it challenging to use for training AI models. To address this challenge, there is a need for better data management and data cleaning techniques that can extract relevant information from unstructured data and ensure the accuracy and completeness of the data.
Another challenge is the need for AI algorithms to be interpretable and transparent. In some applications, such as healthcare or finance, it is essential to understand how an AI algorithm is making decisions. Black-box algorithms that provide little insight into their decision-making process can be problematic when they make erroneous or biased decisions. Therefore, there is a need for explainable AI techniques that can provide transparency and insights into how the AI model is making decisions.
The real world is also characterized by constant change, uncertainty, and complexity. AI models that perform well in a controlled environment may not be able to handle the unpredictable and dynamic nature of real-world scenarios. Therefore, there is a need for AI algorithms that can adapt and learn from new and unexpected situations, such as changes in consumer preferences, market trends, or global events.
Finally, AI needs to be practical and cost-effective. Real-world applications of AI must provide a return on investment that justifies the cost of development and implementation. AI models must be scalable, easy to use, and integrate with existing systems to be practical in a real-world setting.
To overcome these challenges, researchers and practitioners are developing new approaches to AI that can address the specific needs of real-world applications. For example, transfer learning techniques can be used to adapt pre-trained AI models to new domains with limited data. Explainable AI methods, such as rule-based systems, can provide transparency and accountability in decision-making. Reinforcement learning algorithms can enable AI models to learn from feedback and adjust their behavior over time. Additionally, cloud-based AI services can provide cost-effective and scalable AI solutions for businesses of all sizes.
In conclusion, AI has the potential to transform various sectors of the economy and create new opportunities for businesses to improve efficiency and increase profitability. However, to realize this potential, there is a need for AI that can operate in real-world environments, with real-world data, and real-world constraints. Addressing the challenges of applying AI in the real world will require ongoing research and development efforts, as well as collaboration between academia, industry, and policymakers. With the right approaches and solutions, AI can be a powerful tool for solving real-world problems and advancing human progress.